Blog
Algorithmic Bias in Credit Scoring: How to Limit their Effect?
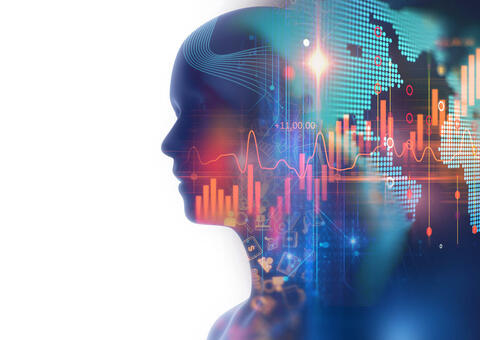
Credit scoring has the potential to improve access to capital and financial inclusion around the world. As financial institutions begin to have access to different types of data, the variety of credit scoring mechanisms have increased. One way that financial institutions have kept track of these alternative data and found ways to understand them is through the use of algorithms and artificial intelligence. In a world with massive data, algorithms help financial institutions make decisions. Innovative methods for credit scoring have the potential to expand access to capital and other financial services for marginalized communities. However, their creation must be intentional and transparent to account for the possible biases that may arise from biased data or biased algorithms.
Many believe that in order to remove this bias, algorithms should not track things like race and gender. They believe that disaggregated data will deter these algorithms from being biased, however, this is not the case. Even if race and gender are not explicitly tracked, the algorithm can still pick up on the bias because of the structural and systemic nature of these biases.
Proxy discrimination happens when a legally prohibited characteristic (like gender or race) can be predicted by other non-suspect data intentionally or unintentionally. There are many proxies for gender, such as:
-
Occupation;
-
Places where people shop;
-
Items that people spend money on;
-
The types of healthcare people access;
-
The type of apps that people download;
-
The type of music that people listen to.
Removing a variable does not eliminate bias. In fact, this blindness often makes it harder for companies to identify and prevent bias since they cannot keep track of it. As long as humans are vulnerable to biases, algorithms will be vulnerable too.
Champions of innovative scoring have highlighted five strategies to help financial institutions minimize the effects of bias when it comes to credit scoring, and to improve overall algorithmic hygiene. The mnemonic device for these strategies is REACT:
-
Regulation – Regulatory capacity should be built to ensure the models that financial institutions are using are adapted to the customers and that these models enable the rest of the strategic criteria – explainability, accountability, collaboration, and transparency. This way, frameworks can be developed and tested and then evaluated by an outside source.
-
Explainability – All decisions made by the algorithm should be easily explainable to the potential borrower. The decision should be simple and straightforward.
-
Accountability – Financial institutions should hold themselves accountable for minimizing bias in their algorithms by setting financial inclusion key performance indicators.
-
Collaboration – As innovative scoring continues to grow, financial institutions should share knowledge on best practices surrounding financial inclusion.
-
Transparency – Credit service providers should understand the frameworks that are being used and be able to explain the data used, where the providers obtained the data, why they are using the data and the basis for the credit scoring decision. Consumers should have access to the steps of the lending process, what is involved in decision-making, and the rationale for why credit decisions are made.
There are many champions that we can look to who are doing a great job of mitigating gender bias in the financing industry.
-
African Academic Network on Internet Policy (AANOIP) is a network for interdisciplinary scholarly engagement and discussion on the State of the Internet, related policies and regulatory regimes in Africa. As a think tank AANOIP publishes its findings on best practices related to regulation and has championed the need for inclusive algorithmic design.
-
Wakandi – Launched the Credit Association Management System in Africa to digitize the way informal financial groups operate, helping to digitize these financial spaces and provide more women with an official identification system where their financial transactions can be tracked. By digitizing informal financial groups, Wakandi has created a method to increase the amount of information available to input into an algorithm, diversifying data and creating access.
-
The Algorithmic Justice League - The Algorithmic Justice League is an organization that combines art and research to illuminate the social implications and harms of artificial intelligence.
-
The Alliance for Inclusive Algorithms - The < A+ > Alliance, founded 2019, is a global coalition of technologists, activists and academics who champion Inclusive AI and Affirmative Action for Algorithms so that we come closer to creating gender and racial equality in a world where machine learning does not wire an already biased system into our future.
While algorithms have a lot of potential for growing financial inclusion, they are not perfect and may contain the biases of the humans that create them. As such financial institutions must ensure that they are not over-relying on this or any other technology in order to minimize bias, and must perform due diligence utilizing complementary human and computational means while working to improve their algorithmic hygiene.
-------------------------------------
Citations:
AFD Supports Digital Financial Inclusion, Especially for Women, Over the African Continent | Agency Francaise of Development
Better Algorithms and Open Data Frameworks Needed For Inclusive And Diverse Online Content | African Academic Network on Internet Policy
Credit Scoring Guideline | World Bank
The Stories Algorithms Tell: Bias and Financial Inclusion at the Data Margins | Center for Financial Inclusion
Women’s Digital Financial Inclusion in Africa | G7 and the Bill and Melinda Gates Foundation
Proxy Discrimination in the Age of Artificial Intelligence and Big Data | Iowa Law Review